Our group's research currently focuses on the following topics:
Sex-specific methylation detection based on nanopore sequencing.
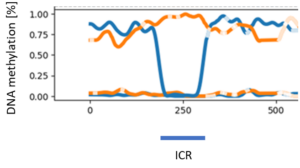
Automated detection of RNA modifications using machine-learning techniques
More than 170 post-transcriptional modifications have been discovered in various RNA species, several of which are associated with multiple human diseases when aberrant. We aim to analyze RNA signal data sets obtained by direct sequencing technology. In detail, our goal is to combine novel machine learning technique with new algorithms that allow both RNA sequence and RNA base modification identification with high accuracy. Additionally, we want to classify the detected modified region with one of the possible post-transcriptional modifications to obtain an accurate RNA sequence pipeline that can be used in diagnostic in the future.
Collaboration partners: Prof. Dr. Mark Helm
Sequencing of wastewater samples as a pandemic early warning system
Metagenomic studies benefit from third generation sequencing, as the long reads allow a more precise differentiation of metagenomic species, and analysis can be done in real-time, producing results within minutes, while the run is still active. One example is the sequencing of regional wastewater plant specimens with the goal to identify the species and resistance genes contained in the sample. This is done partly using already established database classification pipelines and meaningful integration of de novo assembly approaches is underway.
Cooperation partners: Sequenzierkonsortium
Real-time analysis using Nanopore-Sequencing
We are working on a nanopore real-time transcriptional analysis pipeline, that allows differential gene and transcript expression analysis on a global and single gene scale for direct RNA sequencing and barcoded or non-barcoded cDNA. The GUI will help the user to configure and initiate the sequencing analysis and provides a collection of ready-to-download plots for each analytical step. A next-flow-driven preprocessing pipeline allows the alignment, assignment, and quantification of reads in batches on an iterative basis. The pipeline detects newly created data on the output folder of a sequencing experiment and additively combines preprocessed and newly created data. After each iteration, the preprocessing of data can be temporarily paused to analyze the by the time-preprocessed data. After the intermediate analysis is completed, the preprocessing can be easily resumed. The tool is embedded in a docker container to allow an uncomplicated and standardized installation. A preprint of the software-package can be found here
Collaboration partners: Dr. Tamer Butto, Prof. Dr. Mark Helm
Funding: Landesforschungsinitiative Reality
Chromatin conformation capture analysis allows for the investigation of the spatial organization of chromatin in the nucleus
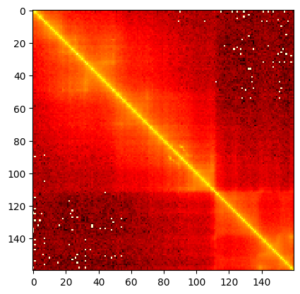
Application of nanopore-sequencing for genomic and epigenomic studies
Nanopore-sequencing (Nanopore-seq) is a rising long-read sequencing technology that provides relatively cost-friendly and rapid products to sequence nucleic acids. Currently, our lab is working in close collaboration with the human genetic diagnostic facility and has applied nanopore-seq for a variety of applications such as SARS-CoV-2 variant detection in line with our Covid-19 Sequencing Initiative, the sequencing and detection of human monkeypox, cancer panel analyses for identification of SVPs of risk, bacterial genome sequencing and sequencing of wastewater samples for pandemic prediction and monitoring. Our focus is to establish novel pipelines and working strategies to sequence and analyse long-read data and apply them routinely in a variety of ongoing and upcoming research projects.
Collaboration partners: Dr. Jennifer Winter, Prof. Dr. Susann Schweiger, Dr. Matthias Linke
Deciphering the epigenetic basis of resilience
This study examines the molecular mechanisms regulating neuronal function in stress-susceptible and resilient mice following chronic social defeat (CSD) by comparing the transcriptomes of activated cells in selected brain regions using RNA-seq. In addition, using the assay for transposase-accessible chromatin followed by high-throughput sequencing (ATAC-seq) and bisulfite sequencing (Bis-seq), we will monitor the global changes in chromatin accessibility and DNA methylation signatures that alter the functional states of neurons in response to stress.
Collaboration partners: Dr. Jennifer Winter, Prof. Dr. Beat Lutz
Funding: CRC 1193 Resilience (http://crc1193.de/)
Using multi-omics integration to explore the molecular background of stress resilience
Resilience is the ability to cope with stress or to quickly recover to a pre-crisis state after being exposed to extreme stress. As the human mental status is highly diverse, there are presumably many different molecular mechanisms underlying resilience. The main goal of this project is the identification of common molecular patterns between resilient individuals by analyzing and integrating various omics levels, e.g. transcriptomics, proteomics, methylomics and metagenomics. By subsequently selecting important features within the large amount of data, we aim to give a less complex view of the dynamic process of resilience. This work is in collaboration with the Leibniz Institute for Resilience Research (LIR) (https://lir-mainz.de/). The majority of the underlying data sets originate from the MARP (https://marpstudie.de/) and LORA (https://lora-studie.de/) studies from the LIR.
Collaboration partners: Prof. Dr. Raffael Kalisch
Funding: Reality Initiative
BIG data integration of genetic and epigenetic variations in neurodegenerative diseases
To gain a better understanding of the global mechanisms underlying neurodegeneration we use supercomputing facilities and recently developed High-Performance Computing methods in multivariate Genome Wide Association Studies
(GWAS) for the extraction of global patterns. Analysis includes genetic as well as epigenetic and transcriptional aspects, underlying neurodegenerative diseases i.e. Alzheimer’s, Parkinson’s and Huntington’s disease. Via a trans-Omics evaluation followed by in silico modeling we hope to extract core (biochemical) networks across multiple omic-layers (Genome, Transcriptome, Methylome).
In other words, the goal of this project is to integrate data from different sources (genomes, transcription datasets, DNA methylation and others) in order to determine which information is most relevant in order to explain the phenotypes observed in neurodegenerative diseases such as Alzheimer’s Disease. This will hopefully lead to the identification of genes or pathways which are involved in disease formation. Available genomes of control and Alzheimer patients (Alzheimer sequencing project) will help to find variations in coding regions, promoters and regulatory regions (whether SNP or larger variants). RNA-seq will provide both
information about expression and possible mutations in structural expressed regions of the genome. As such, noncoding RNA could be of special focus as mutations in protein-coding regions are rare. Epigenetic data will finally bring information on possible regulatory mechanisms of genes.
Collaboration partners: Prof. Dr. Dorothee Dormann, Prof. Dr. Susann Schweiger
Discovery of polygenic adaptation patterns in Chironomus riparius
In cooperation with the Senckenberg Biodiversity and Climate Research Center Institute Frankfurt am Main, we are currently researching the genetic effects of rapid adaptation caused by selection. In an ongoing experiment, a C. Riparius population is set under selection pressure by only using the early emerging midges for continuing the line. We then employ sequencing analysis of these adapted individuals to test the polygenic adaptation. Ws are aiming at uncovering the pattern of those genes that have changed together under selection pressure.
To this end, it is of great importance to secure a solid method for variant calling, which mostly makes use of machine learning. this, we are testing and comparing different variant calling tools for both individual and pooled sequencing data such as GATK, DeepVariant, Population and CRISP.
Secondly, with the help of unsupervised machine learning (also better known as data mining), we are interested in finding the pattern of polygenic adaptation by means of pattern recognition and clustering.
Collaboration partners: Prof. Dr. Markus Pfenninger
Funding: Research Center for Emergent Algorithmic Intelligence (Emergent AI Center)
Characterization of gut microbiota composition using 16S rRNA sequencing
Sequencing of the 16S ribosomal RNA marker gene (16S rRNA) provides a cost-effective method to characterize the bacterial composition of biological or ecological samples. In experimental animal studies, this approach allows investigation of the impact of factors such as pharmacological treatment or diet on commensal microbiota. Another crucial research question is whether shifts in gut bacterial composition are associated with disease phenotypes. In ongoing collaborations with experimental groups, we focus on the bioinformatical and biostatistical analysis of 16S sequencing data. This requires applying ordination techniques that can handle distance measures appropriate for ecological data such as principal coordinates analysis or correspondence analysis. Furthermore, no consensus on the optimal statistical technique for differential abundance analysis exists. Therefore, this decision needs to be based on considerations such as sample size, experimental setup, and specific research questions.
Collaboration partners: Prof. Dr. Beat Lutz, Prof. Dr. Christoph Reinhardt